Decoding the chess moves of Snowflake and Databricks – Business
As customers try to “get artificial intelligence right,” the need to rationalize siloed data becomes increasingly important.
Data practitioners could put all their data eggs into a single platform basket, but that’s proving impractical. As such, firms are moving to an open model where they maintain control of their data and can bring any compute engine to any of their data.
While compelling, the capabilities to govern open data across an entire estate remain immature. Regardless, the move toward open table formats is gaining traction and the point of control in the data platform wars is shifting from the database to the governance catalog.
Moreover, as data platforms evolve, we see them increasingly as tools for analytic systems to take action and drive business outcomes. Because catalogs are becoming freely available, the value in data platforms is also shifting toward tool chains to enable a new breed of intelligent applications that leverage the governance catalog to combine all types of data and analytics, while preserving open access. Two firms, Snowflake Inc. and Databricks Inc., are at the forefront of these trends and are locked in a battle for mindshare that is both technical and philosophical.
In this Breaking Analysis, we take a look back at what we learned from this year’s back to back customer events from the two leading innovators in the data platform space. We’ll share some Enterprise Technology Research data that shows how each firm is faring in the other’s turf and how a new application paradigm is emerging that ties together data management, open governance and of course, AI leadership.
Open formats shift the value in data platforms
Let’s start by looking at how the landscape of data platforms is shifting under our feet. We see five areas that underpin a platform shift where systems of models will, we believe, ultimately come together to define the next architecture for intelligent apps.
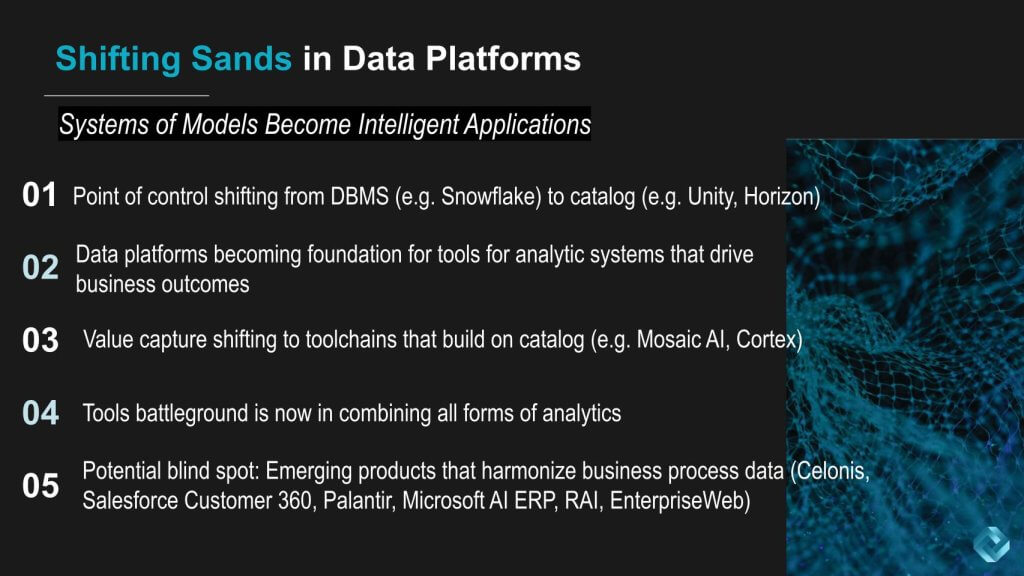
Shifting point of control. As we previously mentioned, the point of control began to shift last year when Databricks announced its Unity Catalog. In response, Snowflake this month open-sourced its Polaris technical metadata catalog. Databricks then acquired Tabular (founded by the creators of Iceberg) and subsequently open-sourced Unity.
At the 2023 Databricks Data + AI Summit, Matei Zaharia, the creator of Spark and co-founder of Databricks, introduced an enhanced version of its Unity Catalog. This moment was pivotal, signaling a shift in control over the system of truth about your data estate from the DBMS to the catalog.
Traditionally, the DBMS has had read-write control over data. Now, the catalog is set to mediate this control. This does not eliminate the role of the DBMS; rather, a DBMS-like execution engine attached to the catalog will manage read-write operations. However, this engine can be a new, embedded, low-overhead, and low-cost SKU. This distinction is crucial in determining who maintains the point of control.
Data becomes a feeder for applications that act. Let’s come back to the list above and talk about Nos. 2, 3 and 4 here. We’re envisioning an application shift where data platforms increasingly inform business actions. Value is shifting and will continue to shift toward tools and workflows that build on and leverage the governance catalog. Here we’ve cited both MosaicAI, an offering that comes out of Databricks’ acquisition of MosaicML last year; and Cortex, Snowflake’s machine learning/AI managed service.
Further elaborating, today data platforms have mostly been about building standalone analytic artifacts, what some people call data products, whether it’s dashboards, ML models, maybe even as simple as refined tables. Now we’re getting to something slightly more sophisticated in the form of retrieval-augmented generation-grounded Gen AI models. By RAG-grounded, we mean there’s a retriever and vector embeddings that output simple request-response artifacts.
We believe we’re moving toward systems that drive a business outcome. Examples include nurturing a lead down a funnel or providing expertise to a customer service agent as to how to guide a more effective response to a customer online or to forecast sales and then drive operational planning. These are more sophisticated workflows that include both a supervised human or a supervising human and an agent that’s figuring out how to perform a set of tasks under that human supervision to drive some sort of outcome.
Wild card: the so-called semantic layer. Now, coming back to the final point on the graphic above, there’s a potential blind spot brewing further up the stack. We’ve discussed, for example, the Salesforce Data Cloud and its customer 360 approach, where applications such as Salesforce embed the business logic and Salesforce and others on the list have harmonized the business process data, which is a piece that both Snowflake and Databricks appear to be missing or perhaps we should say are relying on the ecosystem to deliver.
Why is this a potential disruptor to the current leaders?
Because when you’re building these analytic systems to drive business outcomes, they need the context of what is the state of the business, what happened in the business in order to determine what should happen next. If you just have a data lake that has 10,000 or 100,000 tables or however many it is, you don’t know what 500 tables have some parts of all the attributes that define the context with, for example, a particular customer — or beyond this, how a specific customer relates to a sales process or a service process. None of today’s data platform firms has that capability today.
When you probe the leaders on things such as a graph database, which could harmonize all this context, our interactions suggest they see this either as a niche, or as something the ecosystem should deliver. Or in some instances, we’ve uncovered clues that they’re working on graph databases to add capabilities to the catalog.
But even if they build this knowledge graph that has the people, places and things in the business, it doesn’t yet give them as rich a map of the state of the business possessed by firms such as a Celonis Inc., which mines from all the application logs; or Microsoft Corp. is building with its AI enterprise resource planning effort as part of the power platform in Dynamics. Palantir Technologies Inc. also has something like this, as does EnterpriseWeb LLC and RelationalAI Inc. These firms are building technologies that should make it easier to build that sort of capability.
The point is the tool chains are there, but when we say they build on a catalog, that catalog itself has to grow in sophistication to make sense out of the business so that the tools know how to consume it. If the application vendors play this role, then either we risk more data silos or possibly disruptions to today’s data platforms.
Databricks and Snowflake move into each other’s territory
Databricks in database – Survey data shows strong momentum for Lakehouse
In the past we’ve talked about how Snowflake had the lead in database and Databricks was having to play catch-up in that regard. But Databricks indicated at its Data + AI Summit that its Lakehouse offering was the fastest-growing product in the company’s history, and stated that it had surpassed a $400 million run rate. So let’s take a look at some of the ETR data to see what it tells.
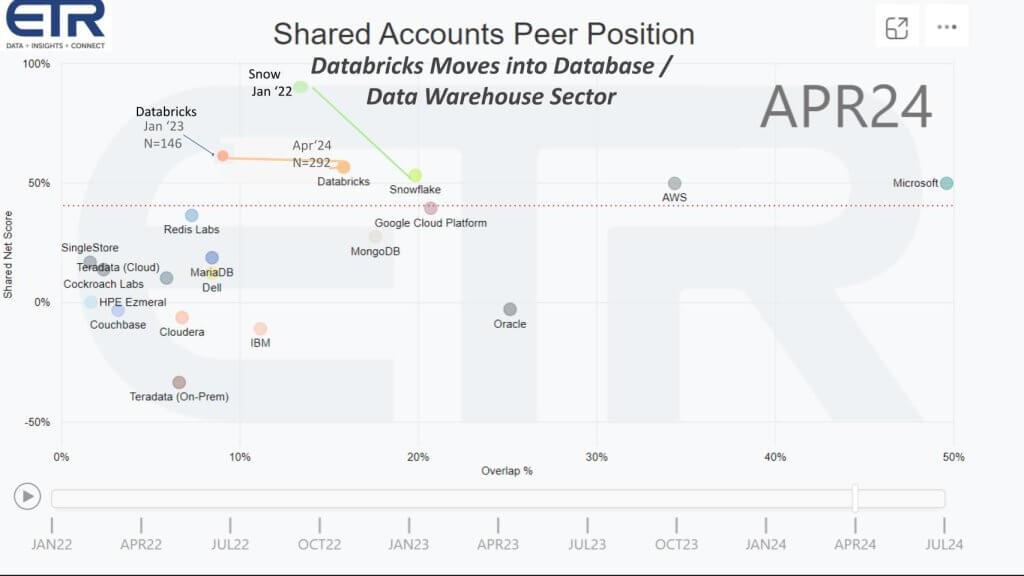
The graphic above is from the April survey of more than 1,800 information technology decision-makers or ITDMs. Net Score or spending momentum is shown in the vertical axis. That’s a measure of the net percentage of customers in the survey that are spending more on a platform. It is derived by subtracting the percentage spending less from the percentage spending more.
The horizontal axis is Overlap or pervasiveness in those roughly 1,800 accounts. That red line at 40% on the vertical axis represents a highly spending momentum. This data cut is for the database and data warehouse sector.
The key point we want to make is that Databricks first showed up in the survey back in January 2023 and is showing both elevated spending velocity and impressive penetration in the dataset. Note that its Ns have increased from 146 in January of 2023 to 292 in April 2024. So it has made major moves to the right while holding momentum on to the vertical axis.
You can see that Snowflake was up into the stratosphere (80%+) on the Y axis in January of 2022. Snowflake has also made strong moves to the right as well, but its momentum has decelerated, consistent with the deceleration in its revenue growth rate, which is tracking in the 25% to 30% range. The point is that Databricks actually showing up more prominently than one might expect. Its Lakehouse revenue is likely growing significantly faster than its overall revenue which we believe has been tracking over 50%.
Snowflake in ML/AI – Survey data shows strong penetration for Snowflake
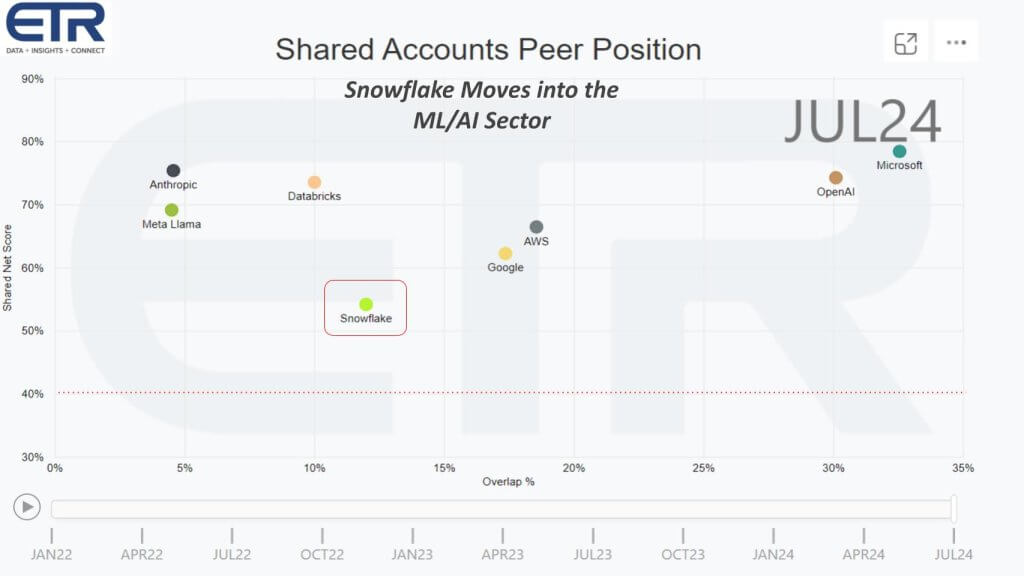
Now let’s flip the script and focus on the sector that has historically been the stronghold of Databricks in an area where Snowflake was really not considered a player. Above we’re showing the same dimensions but isolating on the ML and AI segment of the survey. Net Score or spending velocity on the Y axis and pervasiveness on the X axis. Snowflake was just added to the sector in the survey that’s in the field today, so it’s late June and the survey will close in July. So this is preliminary data, but you can see the early returns above for an N of more than 1,500 accounts.
The data shows Snowflake, while much lower than Databricks on the vertical axis is: 1) Above the 40% magic line; and 2) Further to the right than Databricks, indicating a strong adoption of its new AI tooling.
Our view is it’s significant that Snowflake did a really good job with Cortex, embedding generative AI capabilities. Our take is this is a natural outgrowth of two of its strongest personas, data engineers and data analysts, making it dead simple for these folks to use gen AI capabilities within, for example, stored procedures in the database.
So it up-leveled the capabilities of their personas through what they do best, which is make it really easy to use new functionality.
Databricks is high on the Net Score or spending momentum likely because it did such a good job up-leveling the data scientists, data engineers and ML engineers as extensions of their existing tool chains. That was what it did that impressed us, for instance, instead of making gen AI an entirely new tool chain, ML engineers became large language model ops engineers.
This was enabled because MLflow, which is Databricks’ standard for operational tracking of models, expanded to encompass tracking LLMs. Then Unity absorbed that tracking operational data so that, again, the existing personas were up-leveled to take advantage of the new technology. Our belief is since the data scientists, data engineers and ML engineers were the natural center of gravity for gen AI spending, Databricks harnessed that dynamic effectively to maintain its accelerated momentum.
Control point shifts to the catalog
Below is a chart that Databricks put up at its event last week showing all the different data platforms that Unity connects to, the different types of data and capabilities it offers, and the open access to a variety of engines. The basic ideas is to bring any of these processing engines to the data and have them all governed by Unity.
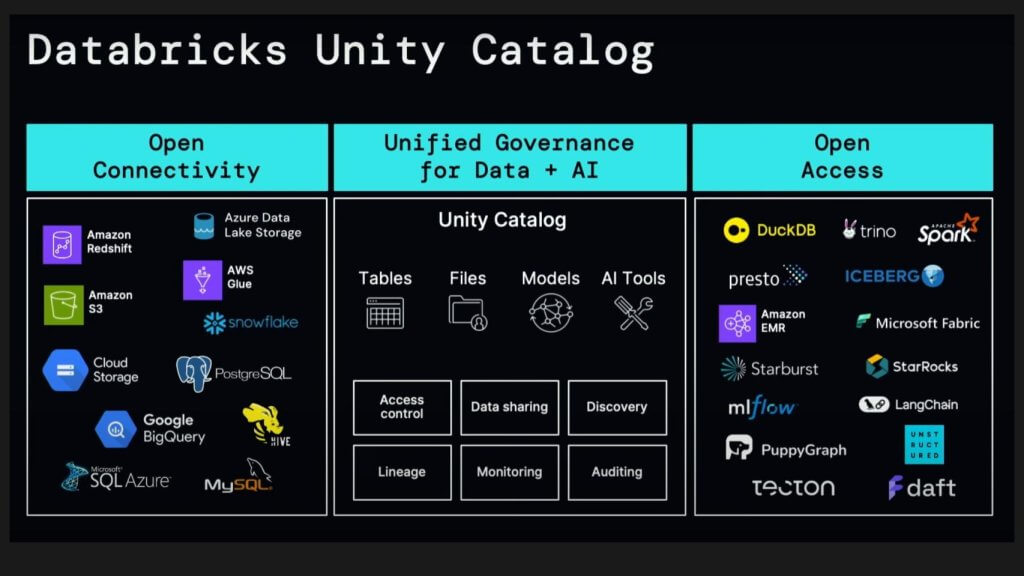
Coming back to our premise that the point of control is shifting to the catalog: Not the point of value necessarily, although Snowflake is trying to hang onto that value with Horizon. The point is because much of the core data governance function in the catalog is being open-sourced, the value, we believe, is shifting elsewhere, which we’ll discuss later.
Working backwards from customer needs
Databricks Chief Executive Officer Ali Ghodsi shared the graphic below to summarize the sentiment of the customers. He points out that every chief information officer, board member, CEO, leader, you name it, wants to get going on AI, but they’re also leery of making mistakes and putting their companies in jeopardy from a legal and a privacy standpoint. So they need governed AI, and as we said upfront, they also recognize that a fragmented data estate is a recipe for bad AI, high costs, low value and basically failed projects.
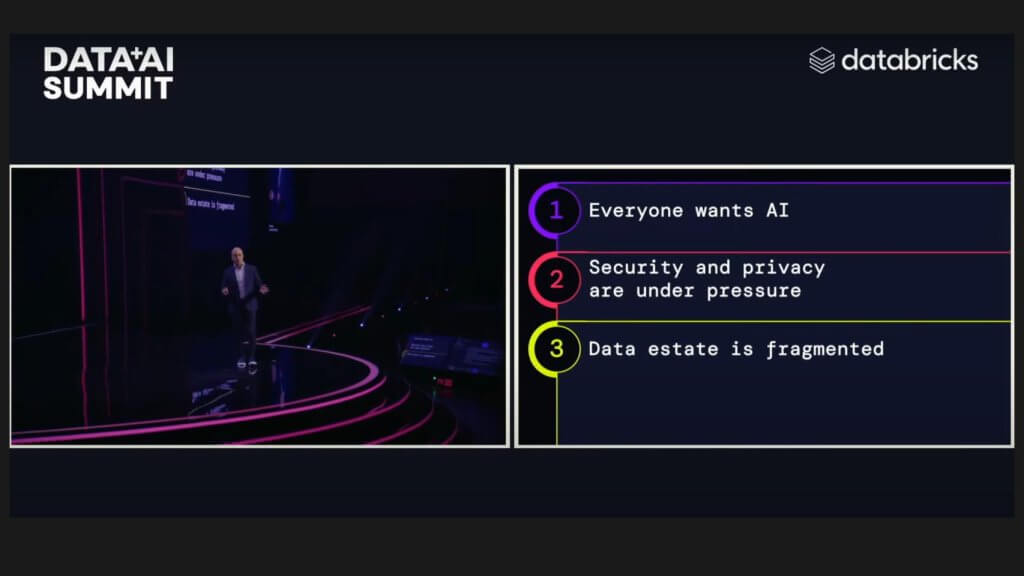
The vision that Databricks put forth at its Data + AI Summit was compelling. One of the comments Ghodsi made was, “Stop giving your data to vendors… even Databricks, don’t give it to us either… don’t trust vendors.”
Stop giving your data to vendors… even Databricks, don’t give it to us either… don’t trust vendors.
Ali Ghodsi, CEO, Databricks, 2024
So a little fear-mongering there, clearly you have to trust vendors on many things, but he’s saying don’t put all your data into a vendor-controlled platform (for example Snowflake), rather keep it open and control it yourselves. And use proprietary tools from us (Databricks), or other engine providers (including Snowflake) to create value from the open data.
The vision is control your own data and be able to bring any engine to the data and apply the best engine for the job that you’re trying to accomplish. Basically: Use the right engine for the right job and may the best engine win.
This message resonates with customers. However, when we talk to customers, they tell us they love the vision, but when we talk about governance, the answer we typically get is, “We’re still trying to figure that out.” Do they go with Unity? Do they go with Polaris, Horizon, something else? Most customers are still trying to determine this. Part of the reason is this world of governance is evolving very rapidly.
The next section of this post that really underscores this fact.
Matei Zaharia’s mic drop moment
Open-sourcing Unity in front of 16,000 friends
One of the big questions going into Databricks Data + AI Summit was: How would Databricks respond to Snowflake’s move of open sourcing Polaris, its technical metadata catalog? As we said in our Snowflake Summit analysis, we wanted to see what it would do with Unity and Tabular. Zaharia answered the Unity question by making the product open under the Apache 2.0 license. This was a dramatic moment at the event and if you didn’t witness first hand, watch the clip below.
Why open-sourcing Unity is not necessarily a nail in Polaris’ coffin
This moment was like a tennis match where, when the camera is focused on the audience, you watch the heads snapping back and forth. At Snowflake Summit, we thought open-sourcing Polaris would sever Unity’s connection with Iceberg tables. Then as you saw the next week, everyone thought Databricks open-sourcing Unity as a rich operational and business catalog would sever Polaris’ hold over Iceberg data.
Then when the dust started to clear, it became clear that it wasn’t so simple. Because what happens is, and this wasn’t as clear as it should have been coming out of Snowflake, the Snowflake-native Horizon operational and business catalog that is part of the Snowflake data platform actually federates all the governance information with Polaris.
So all your security, privacy and governance policies are replicated and synchronized with the Polaris catalog so that you can have unified governance of your Snowflake data estate and your Iceberg data estate — where, to be clear, when the data is in Snowflake, including managed Iceberg tables, third parties can read that Iceberg data. They can’t write it.
Now Polaris governs the external tables that anyone else can read and write and Snowflake can read, but those external tables now have unified governance. So if you’re a Snowflake shop and you have Iceberg tables, you have a comprehensive governance solution.
What if you are a Delta shop and you’re using Unity?
In this case, any engine can read and write Delta tables. So that’s an advantage for Databricks. But third-party tools only have read access to Iceberg tables. Many people don’t realize that Unity is not yet able to mediate write access to Iceberg tables. That’s a problem and that’s why we think Tabular, started by the creators of Iceberg, was part of such a bidding war and ultimately was snapped up by Databricks.
So right now it’s game on and the stakes keep getting higher.
Can Databricks make Delta and Iceberg data interoperable?
The other big chess move occurred during Snowflake Summit. Just as Snowflake’s co-founder Benoit Dageville was stepping on the stage to present his keynote, Databricks dropped a press release announcing that it was acquiring Tabular. You may recall we had Ryan Blue, the CEO of Tabular, on a previous Breaking Analysis. George has also featured Blue on his program and we’ve discussed all these different formats including Delta tables, which is the default format in Databricks and very widely adopted.
Listen below to Blue talk about the difficulty in translating between multiple formats. It’s worth mentioning, just to emphasize, he made these comments before Iceberg was in acquisition play. This is unvarnished and is not PR-scrubbed.
Let’s translate the implications here. Databricks nows has under its control the creators of Iceberg, meaning it has the technical talent to make Delta and Iceberg interoperable and as seamless as possible.
But based on what we heard Blue say, it’s not easy, although it completely changes the dynamics. The problems Tabular was working on really extended beyond making the table formats function seamlessly, to adding the governance capability. Tabular, we believe, was really working on a catalog with a sophisticated policy engine for advanced governance. It became pretty clear when Blue was onstage in Ali’s discussion of the objectives of the Tabular acquisition that the goal now is redirecting all the brains of that 40-person Tabular team more toward interoperability with Delta format.
In other words, adding a policy engine is no longer necessary because that’s going to be subsumed by Uniform. Uniform is a capability introduced by Databricks that allows data stored in Delta Lake to be read and written as if it were in Iceberg or Hudi formats, without the need for making copies of the data. This is a very attractive scenario for Databricks (if it works), eliminating the need to duplicate data and further support that single version of the truth.
This presents a potential downside for Snowflake, which — rather than seeing Iceberg evolve in the direction of adding the advanced functionality that’s in the proprietary version of Iceberg tables that Snowflake has — could move in the direction of just Delta interoperability.
Of course, given the open-source status of Iceberg, Snowflake can commit resources to provide that capability if it chooses to do so. Our sense coming out of Snowflake Summit was that Snowflake would wait to see if a pure open-source approach could deliver full read/write functions. And if it couldn’t, then customers would choose its managed Iceberg service. But waiting too long could give Databricks a head start that may be insurmountable.
AI remains center stage
Let’s turn our attention to AI. Last year right before the Data + AI Summit, Databricks announced the acquisition of a company called the Mosaic ML for about $1. 3 billion, bringing more AI talent into the company. Databricks has leveraged this acquisition to offer Mosaic AI, a diagram of which is shown below.
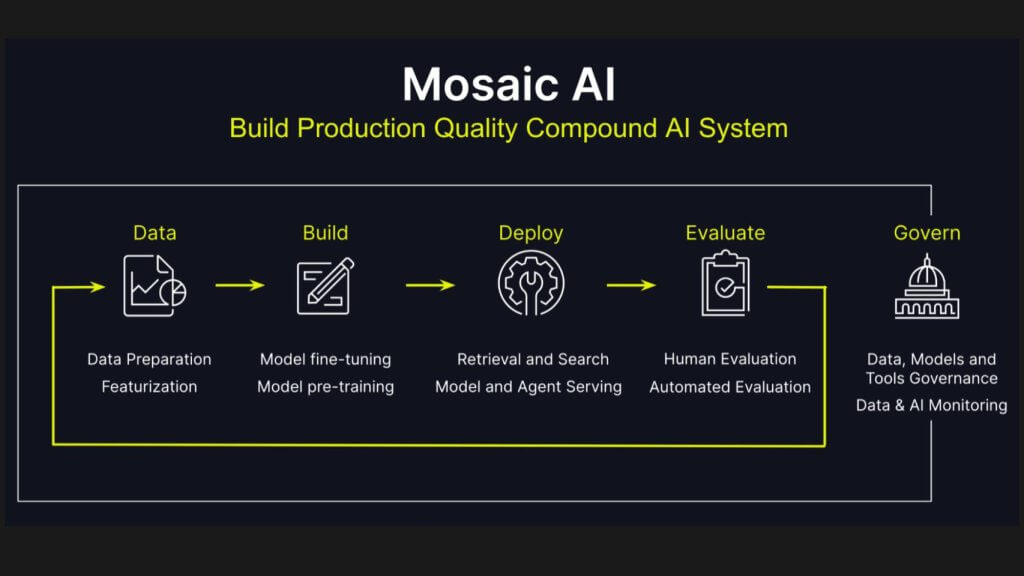
Fifteen months ago, we thought the gen AI wave was something Microsoft would use to try to steamroll Databricks because it was a new tool chain that was disruptive to Databricks. Databricks proved us wrong by doing an astonishing job of up-leveling the personas they had with new capabilities, many of which came from the Mosaic acquisition. Now, what’s key here is that, again, we’re not building standalone artifacts, we’re increasingly building systems of models, each of which has specialized tasks.
What’s crucial about the tool chain is you’re going to optimize how all those pieces work together. That’s not all there yet, but the pieces are coming together. What wasn’t formally announced yet, but came out in deeper discussions, is that Databricks has hired the researcher who created DSPy, which is really a successor to LangChain. LangChain became sort of uncool once everyone heard about DSPy, which is a way of essentially optimizing a full pipeline of specialized models.
This is not something we’ve seen yet from other vendors, including Snowflake. Also, crucially, the evaluation function in this tool chain is critical because when you’re building continually improving models, the evaluation capability is the critical piece that gives necessary feedback to the models to continue learning.
Check out this Reddit thread on some of the plusses and minuses of DSPy.
So when you put all these pieces together, the Databricks Mosaic tool chain is well on its way toward helping customers build very sophisticated compound systems. This notion that you just have GPT-4, an embedding model, a vector database and a retriever, that becomes less useful. Rather, now we’re building much more sophisticated tool chains, which brings us to the next point: When you start aggregating these tool chains together, you get something more interesting.
Building a system of systems: a new application paradigm
Let’s project that out and try to visualize it. Thinking out a few years, we’ll discuss how we see this application paradigm evolving. What we’ve done below is taken that previous slide and created multiples of them to build essentially a system of systems, where different systems are leveraging foundation models and domain- specific models. These feed a new model – an uber model if you will – that can take action.
Coming back to this notion of the sixth data platform, or maybe beyond that, creating a digital representation of your business that reflects on the state of people, places and things, our Uber-for-the-enterprise metaphor, where the system of analytics can inform the top-level system. And that top-level system is agentic, meaning an intelligent system that’s able to work autonomously, making decisions, adapting to changing conditions in real time, and taking action with or potentially even without human interaction based on the specific use case.
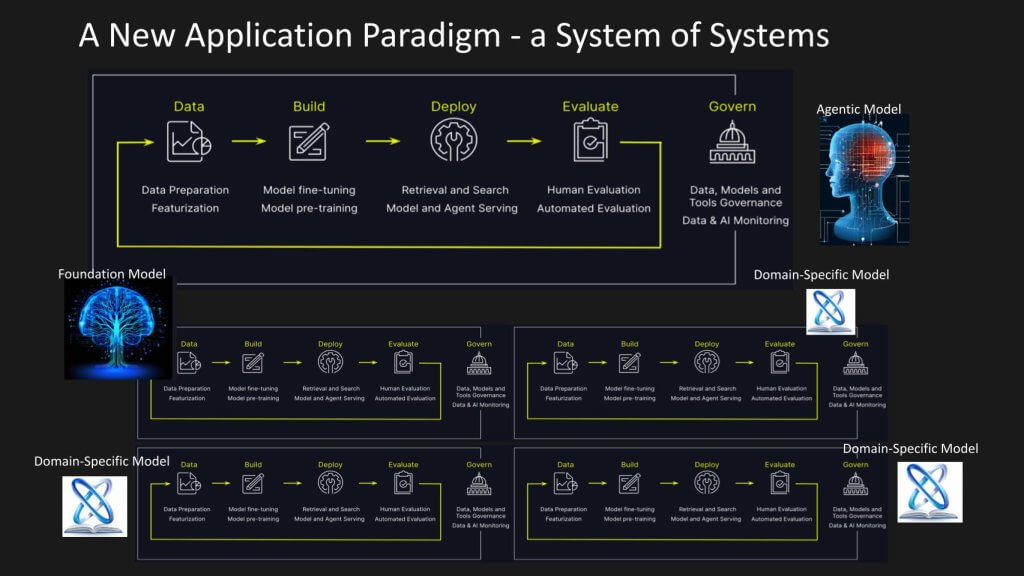
An Amazon example of what’s possible
Let’s take an example of what this might make possible sometime in the future with a frontier vendor. We’ve spoken to Amazon.com Inc.’s — not Amazon Web Services Inc.’s but Amazon.com’s — head of forecasting, Ping Xu. It has taken an “agent of agents” approach that’s really leading edge. What Amazon did is take about 15 years of sales history so that it now has a forecast that can extend five years into the future down to the SKU level for 400 million SKUs, including products that it hasn’t seen yet.
When it gets to the point where it can forecast with reliable precision, it then has a bunch of planning agents that can be coordinated based on that forecast. The scope of those planning agents ranges from how should it build out their fulfillment center capacity, how to configure it, what it should order from each supplier, how to feed supply across the distribution centers, the cross docking, all the way to what gets picked, packed and shipped.
The point of that is you have a system of agents now that are trained together to figure out an optimal set of plans, but that are coordinated in service of some top-level objective — for example growth, profitability, speed to delivery, and the like, all within local constraints for each of the agents. This represents a system of systems that serves to drive a business outcome and that needs very advanced tools that don’t fully exist yet from merchant mainstream vendors. However, a firm such as Amazon is showing the way for what’s going to become possible, and that’s where we see value being created in the future.
Recapping the future of data platforms and predicting next steps
Let’s summarize and end on what we see as the outlook and possible next moves for Snowflake and Databricks, and some of those other players.
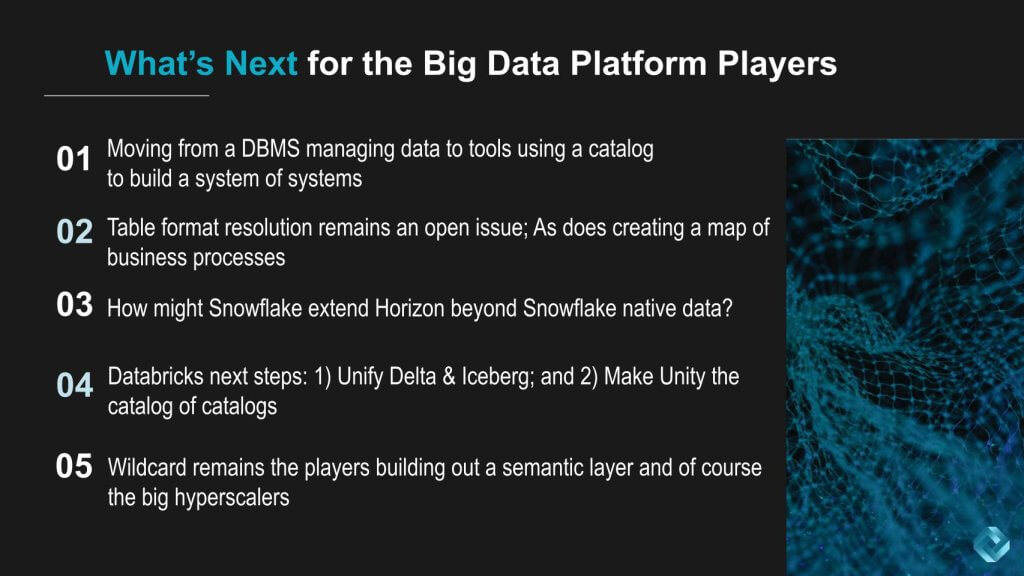
As we’ve said, we’re moving from a database management system-centric control point, where the database is managing the data, to tools using a catalog to build what we just showed you in the Amazon example. This idea is a systems of systems that feeds an uber system, if you will.
The table format resolution remains unresolved. It’s an open issue. We’re going to be watching very closely to see what happens with Databricks, with Tabular, how successful they are at integrating “seamlessly” with Delta, and how Snowflake responds next.
In other words: to what extent Snowflake extends Horizon. Remember, Polaris is the technical metadata catalog that’s open source. Horizon contains the role-based access controls and all the really high-value governance functions. But you basically have to be inside Snowflake to take full advantage of these capabilities. Managed Iceberg tables can take advantage of the format, but that’s again inside of Snowflake.
Where will Snowflake take Horizon? Is it going to go beyond Snowflake native data? We don’t think it has fully decided yet and perhaps it’s going to let the market decide. If open source doesn’t deliver on the promise, then customers might be enticed to stay inside or move more data inside of Snowflake. If open source moves fast, then we’ll likely see Snowflake make further moves in that direction.
We see Databricks’ next steps focused on unifying Delta and Iceberg more tightly to simplify and lower costs for users. It now has the creators of Iceberg to help do that. They deeply understand how Iceberg works better than Databricks does or did.
Many of these acquisitions are designed to pick up talent and this is a good example. You certainly saw that with Mosaic ML. We saw that with Neeva at Snowflake. So the idea to make Unity the catalog of catalogs, that uber catalog that we talked about, is strategically compelling.
The wild card does remain the players that are building out this so-called semantic layer. We talked about folks such as Salesforce with its version of data cloud and firms such as Palantir that are doing some of these interesting harmonization tasks. Of course, the big hyperscalers, we haven’t talked about them in this post – they’re obviously in the mix, so we can’t count them out either.
Possible next moves on the chessboard
Two years ago, most of the discussion was around business intelligence metrics. Then we talked about the enabling technology to make it possible to build a semantic layer with the declarative knowledge graphs from RelationalAI or EnterpriseWeb.
But there are compromised versions today where essentially Salesforce is becoming a hyperscaler with a set of applications and a semantic layer. We’re focused on how the vendors might evolve and try to outmaneuver each other. One example with Snowflake is to take it from being a catalog that’s embedded within the DBMS to a SKU that’s standalone.
Snowflake might, for example, price this capability differently, even if it has the same technology in it. It might be extended so that it uses perhaps something such as Dagster to orchestrate the data engineering workflows that happen beyond the scope of Snowflake. If it does something like this, Snowflake can capture all the lineage data, which is the foundation of all operational catalogs. This is one way Horizon could start extending its reach beyond just what’s happening within the Snowflake DBMS.
We’ll tease these for a later Breaking Analysis episode, but there’s a lot that’s going on because what these vendors are jockeying for really is not just the next data platform, but the next application platform.
It’s the platform for intelligent applications.
How do you see this playing out? What did you learn from this years back to back Snowflake and Databricks events? And what dots are you connecting? Let us know.