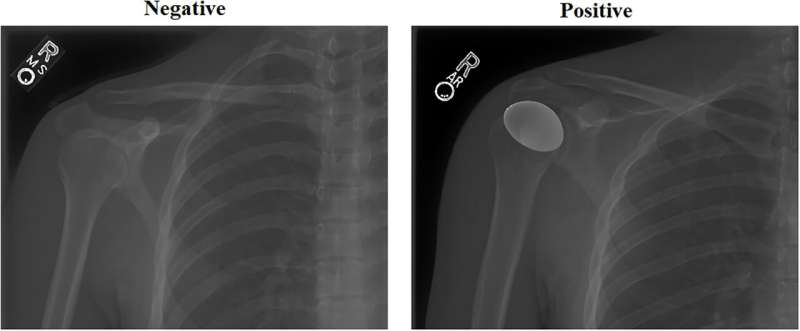
QUT scientists have developed a deep learning framework to detect shoulder abnormalities such as fractures in X-ray images with 99% accuracy to enable clinicians to make correct and speedy decisions in emergency situations.
The findings of the international team led by QUT scientists, were published in the journal PLOS One.
QUT Dr. Laith Alzubaidi, first author of the study, said 1.7 billion people worldwide were affected by painful and debilitating musculoskeletal conditions.
“Musculoskeletal issues can be challenging, especially in emergencies where quick and correct diagnosis and decisions are crucial,” Dr. Alzubaidi, from the QUT School of Mechanical, Medical and Process Engineering and the ARC Industrial Transformation Training Center-Joint Biomechanics, said.
“While deep learning has shown promise in medical decision-making applications, there have been problems of poor performance and lack of transparency in detecting shoulder abnormalities whether they be fractures, arthritis or a deformity in X-rays.”
Dr. Alzubaidi said the process included using a feature fusion technique to combine features extracted from seven deep neural models.
“Machine learning-based classification techniques require fully descriptive features to distinguish between classes to achieve high performance,” he said.
“To accomplish this, we used the feature fusion technique to enhance the results of individual models.
“This technique enables a complete description of the internal information, resulting in a compact representation of fused features, to improve the performance of this task.
“Seven deep convolutional neural networks were individually trained and evaluated to extract features.
“The features extracted from the seven models were fused into one group to train machine learning classifiers.”
Co-researcher QUT Professor YuanTong Gu, Pro Vice-Chancellor and Head of the QUT School of Mechanical, Medical and Process Engineering, said their proposed framework achieved accuracy rate of 99.2%.
“These results outperformed previous computer methods and even human doctors, including three orthopedic surgeons and three radiologists who achieved 79% accuracy,” Professor Gu said.
“The proposed framework has been validated against several potential biases to ensure trustworthy decision-making.
“This tool can provide real-time decisions, which is crucial for such a problem.”
The QUT team comprised Dr. Alzubaidi, Professor YuanTong Gu, Dr. Chun Ouyang, Dr. Asma Salhi, Jinshuai Bai, Dr. Freek Hollman, Adjunct Professor Kenneth Cutbush, and Adjunct Professor Ashish Gupta.
More information:
Laith Alzubaidi et al, Trustworthy deep learning framework for the detection of abnormalities in X-ray shoulder images, PLOS ONE (2024). DOI: 10.1371/journal.pone.0299545
Citation:
Deep learning enables faster, more accurate decisions for treatment of shoulder abnormalities (2024, March 25)
deep-enables-faster-accurate-decisions.html
.
. The content is provided for information purposes only.