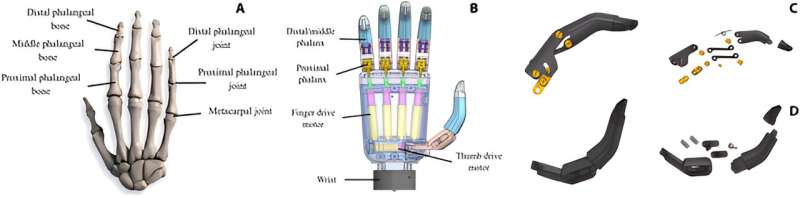
The electromyographic (EMG) signal is the bioelectrical current generated during muscle contraction. It can be transmitted as an input signal to an intelligent bionic prosthetic hand to control hand movements. By increasing the number of signal acquisition channels, richer information about the intention of the action can be captured, thus improving the success rate of the recognition of the intention of the action. However, it is not better to have more acquisition channels.
As the number of channels increases, the hardware system becomes more complex, and the effect of improving the accuracy of gesture recognition gradually decreases, resulting in the control effect reaching a bottleneck.
To address these issues, a team of researchers from Beijing Institute of Technology proposed a method to improve gesture recognition accuracy by virtually increasing the number of EMG signal channels.
The team published their findings in Cyborg and Bionic Systems.
This method extracts amplitude features from EMG signals to represent the contraction intensity of a muscle over time. The absolute values of the intensity differences between channels are then calculated. These difference values are merged with the original data to form new samples with more columns, simulating an actual increase in the dimensionality of the data. This makes use of the implicit coordination information between muscles during movement.
Even if the number of physical acquisition channels is limited, this approach improves recognition accuracy because it does not rely solely on the amount of data directly acquired by the sensor.
To validate their method, the authors compared the accuracy of gesture intent recognition before and after adding virtual dimensions. The accuracy of gesture recognition using EMG signals after the addition of virtual dimensions was improved compared to unprocessed EMG signals. In addition, the greater the number of EMG signal acquisition channels and the richer the EMG signals obtained, the higher the success rate of gesture recognition.
In addition, based on the filtered feature selection approach, the research team introduced a separability metric derived from the dispersion and correlation of the feature set (separability of feature vectors SFV). The SFV value can predict the classification effect before classification is performed and validate the effectiveness of the virtual dimensionality increase strategy in terms of the change in the separability of the feature set.
More information:
Yuxuan Wang et al, A Hand Gesture Recognition Strategy Based on Virtual-Dimension Increase of EMG, Cyborg and Bionic Systems (2023). DOI: 10.34133/cbsystems.0066
Provided by
Beijing Institute of Technology Press Co., Ltd
Citation:
Research proposes virtual-dimension increase of EMG signals for prosthetic hands gesture recognition (2024, April 17)
virtual-dimension-emg-prosthetic-gesture.html
.
. The content is provided for information purposes only.